Document Type
Article
Publication Date
7-4-2019
Abstract
Background
As depression is the leading cause of disability worldwide, large-scale surveys have been conducted to establish the occurrence and risk factors of depression. However, accurately estimating epidemiological factors leading up to depression has remained challenging. Deep-learning algorithms can be applied to assess the factors leading up to prevalence and clinical manifestations of depression.
Methods
Customized deep-neural-network and machine-learning classifiers were assessed using survey data from 19,725 participants from the NHANES database (from 1999 through 2014) and 4949 from the South Korea NHANES (K-NHANES) database in 2014.
Results
A deep-learning algorithm showed area under the receiver operating characteristic curve (AUCs) of 0.91 and 0.89 for detecting depression in NHANES and K-NHANES, respectively. The deep-learning algorithm trained with serial datasets (NHANES, from 1999 to 2012), predicted the prevalence of depression in the following two years of data (NHANES, 2013 and 2014) with an AUC of 0.92. Machine learning classifiers trained with NHANES could further predict depression in K-NHANES. There, logistic regression had the highest performance (AUC, 0.77) followed by deep learning algorithm (AUC, 0.74).
Conclusions
Deep neural-networks managed to identify depression well from other health and demographic factors in both the NHANES and K-NHANES datasets. The deep-learning algorithm was also able to predict depression relatively well on new data set—cross temporally and cross nationally. Further research can delineate the clinical implications of machine learning and deep learning in detecting disease prevalence and progress as well as other risk factors for depression and other mental illnesses.
Recommended Citation
Oh, J., Yun, K., Maoz, U., Kim, T.-S., & Chae, J.-H. (2019). Identifying depression in the National Health and Nutrition Examination Survey data using a deep learning algorithm. Journal of Affective Disorders, 257, 623–631. https://doi.org/10.1016/j.jad.2019.06.034
Copyright
Elsevier
Creative Commons License
This work is licensed under a Creative Commons Attribution-Noncommercial-No Derivative Works 4.0 License.
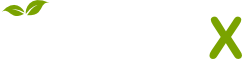
- Citations
- Citation Indexes: 57
- Policy Citations: 2
- Usage
- Downloads: 840
- Abstract Views: 305
- Captures
- Readers: 132
Included in
Community Psychology Commons, Epidemiology Commons, Health Information Technology Commons, Health Psychology Commons, Investigative Techniques Commons, Numerical Analysis and Scientific Computing Commons, Other Computer Sciences Commons, Other Mental and Social Health Commons, Other Psychiatry and Psychology Commons, Other Psychology Commons, Psychiatric and Mental Health Commons, Social Psychology Commons, Theory and Algorithms Commons
Comments
NOTICE: this is the author’s version of a work that was accepted for publication in Journal of Affective Disorders. Changes resulting from the publishing process, such as peer review, editing, corrections, structural formatting, and other quality control mechanisms may not be reflected in this document. Changes may have been made to this work since it was submitted for publication. A definitive version was subsequently published in Journal of Affective Disorders, volume 257, in 2019. https://doi.org/10.1016/j.jad.2019.06.034
The Creative Commons license below applies only to this version of the article.