Document Type
Article
Publication Date
9-6-2024
Abstract
Early recognition of Alzheimer’s disease (AD) and its precursor state, mild cognitive impairment (MCI), is pivotal in interrupting the progression of the disease and providing suitable treatment. Recent development in deep learning techniques has drawn great research attention for improving the efficacy of AD recognition. However, numerous current methods solely utilize data from a single auxiliary domain, limiting their ability to harness valuable intrinsic insights from multiple domains. To cope with the challenge, this paper is devoted to establishing an innovative multimodal medical data fusion model, termed as MMDF, to perform Alzheimer’s disease recognition. Multimodal data including clinical records and medical images are used by the proposed approach, and backbone models are constructed using various data modalities. Specifically, a vision transformer model, which is termed as MRI_ViT, is tailored to recognize AD using brain magnetic resonance imaging (MRI) data. In parallel, a novel multi-scale attention-embedded one-dimensional (1D) convolutional neural network (MA-1DCNN) is devised for analyzing clinical records. Subsequently, these basic models are combined for creating a new data fusion model to recognize Alzheimer’s disease. The experimental results reveal outstanding performance compared with state-of-the-art (SOTA) methods.
Recommended Citation
Chen, J., Wang, Y., Zeb, A., Suzauddola, M. D., & Wen, Y. (2025). Multimodal mixing convolutional neural network and transformer for Alzheimer’s disease recognition. Expert Systems with Applications, 259, 125321. https://doi.org/10.1016/j.eswa.2024.125321
Copyright
Elsevier
Creative Commons License
This work is licensed under a Creative Commons Attribution-Noncommercial-No Derivative Works 4.0 License.
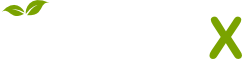
- Citations
- Citation Indexes: 2
- Usage
- Abstract Views: 8
- Captures
- Readers: 14
- Mentions
- News Mentions: 1
Included in
Artificial Intelligence and Robotics Commons, Diagnosis Commons, Health Information Technology Commons, Medical Neurobiology Commons, Nervous System Diseases Commons, Neurosciences Commons, Other Analytical, Diagnostic and Therapeutic Techniques and Equipment Commons, Other Electrical and Computer Engineering Commons
Comments
NOTICE: this is the author’s version of a work that was accepted for publication in Expert Systems with Applications. Changes resulting from the publishing process, such as peer review, editing, corrections, structural formatting, and other quality control mechanisms may not be reflected in this document. Changes may have been made to this work since it was submitted for publication. A definitive version was subsequently published in Expert Systems with Applications, volume 259, in 2025. https://doi.org/10.1016/j.eswa.2024.125321
The Creative Commons license below applies only to this version of the article.