Document Type
Article
Publication Date
1-27-2022
Abstract
Large amounts of autism spectrum disorder (ASD) data is created through hospitals, therapy centers, and mobile applications; however, much of this rich data does not have pre-existing classes or labels. Large amounts of data—both genetic and behavioral—that are collected as part of scientific studies or a part of treatment can provide a deeper, more nuanced insight into both diagnosis and treatment of ASD. This paper reviews 43 papers using unsupervised machine learning in ASD, including k-means clustering, hierarchical clustering, model-based clustering, and self-organizing maps. The aim of this review is to provide a survey of the current uses of unsupervised machine learning in ASD research and provide insight into the types of questions being answered with these methods.
Recommended Citation
Parlett-Pelleriti, C.M., Stevens, E., Dixon, D. et al. Applications of Unsupervised Machine Learning in Autism Spectrum Disorder Research: a Review. Rev J Autism Dev Disord (2022). https://doi.org/10.1007/s40489-021-00299-y
Copyright
The authors
Creative Commons License
This work is licensed under a Creative Commons Attribution 4.0 License.
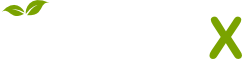
- Citations
- Citation Indexes: 33
- Usage
- Downloads: 381
- Abstract Views: 27
- Captures
- Readers: 131
- Mentions
- News Mentions: 1
Included in
Databases and Information Systems Commons, Diagnosis Commons, Other Analytical, Diagnostic and Therapeutic Techniques and Equipment Commons, Other Computer Sciences Commons, Other Electrical and Computer Engineering Commons, Other Medicine and Health Sciences Commons, Other Psychiatry and Psychology Commons, Psychological Phenomena and Processes Commons
Comments
This article was originally published in Review Journal of Autism and Developmental Disorders in 2022. https://doi.org/10.1007/s40489-021-00299-y