Document Type
Article
Publication Date
6-8-2021
Abstract
Satellite imagery is becoming ubiquitous. Research has demonstrated that artificial intelligence applied to satellite imagery holds promise for automated detection of war-related building destruction. While these results are promising, monitoring in real-world applications requires high precision, especially when destruction is sparse and detecting destroyed buildings is equivalent to looking for a needle in a haystack. We demonstrate that exploiting the persistent nature of building destruction can substantially improve the training of automated destruction monitoring. We also propose an additional machine-learning stage that leverages images of surrounding areas and multiple successive images of the same area, which further improves detection significantly. This will allow real-world applications, and we illustrate this in the context of the Syrian civil war.
Recommended Citation
Hannes Mueller, Andre Groeger, Jonathan Hersh, Andrea Matranga, and Joan Serrat. Monitoring war destruction from space using machine learning. Proceedings of the National Academy of Sciences, 118(23) e2025400118, 2021. https://doi.org/10.1073/pnas.2025400118
Peer Reviewed
1
Copyright
National Academy of Sciences
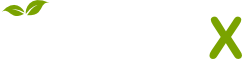
- Citations
- Policy Citations: 4
- Citation Indexes: 25
- Usage
- Downloads: 88
- Abstract Views: 19
- Captures
- Readers: 61
- Mentions
- Blog Mentions: 1
- News Mentions: 2
Included in
Human Geography Commons, Military and Veterans Studies Commons, Peace and Conflict Studies Commons, Remote Sensing Commons
Comments
This is a pre-copy-editing, author-produced PDF of an article accepted for publication in Proceedings of the National Academy of Science, volume 118, issue 23, in 2021 following peer review. The definitive publisher-authenticated version is available online at https://doi.org/10.1073/pnas.2025400118.