Document Type
Article
Publication Date
4-2-2024
Abstract
The recent decades have seen an increasing academic interest in leveraging machine learning approaches to nowcast, or forecast in a highly short-term manner, precipitation at a high resolution, given the limitations of the traditional numerical weather prediction models on this task. To capture the spatiotemporal associations of data on input variables, a deep learning (DL) architecture with the combination of a convolutional neural network and a recurrent neural network can be an ideal design for nowcasting rainfall. In this study, a long short-term memory (LSTM) modeling structure is proposed with convolutional operations on input variables. To resolve the issue of underestimation of heavy rainfall that challenges most of the DL models, a pixelwise modeling approach is adopted to facilitate a stratified sampling process in generating training data points for calibrating models to predict rain rates at locations. The proposed pixelwise convolutional LSTM (CLSTM) models are applied to data on mesoscale convective systems during the warm seasons over the Korean Peninsula. Results show a significant and consistent improvement in prediction skill scores produced by the CLSTM models than a traditional rainfall nowcasting method, the McGill algorithm for precipitation nowcasting by Lagrangian extrapolation, across all considered lead times from 10 to 60 min. Future work needs to reduce the relatively large false positive rates produced by the CLSTM models and their blurring effect in mapping spatial distributions of rain rates, in particular for longer lead times.
Recommended Citation
Y. V. Wang et al., "Nowcasting Heavy Rainfall With Convolutional Long Short-Term Memory Networks: A Pixelwise Modeling Approach," in IEEE Journal of Selected Topics in Applied Earth Observations and Remote Sensing, vol. 17, pp. 8424-8433, 2024, https://doi.org/10.1109/JSTARS.2024.3383397.
Copyright
The authors
Creative Commons License
This work is licensed under a Creative Commons Attribution-Noncommercial-No Derivative Works 4.0 License.
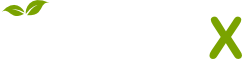
- Citations
- Citation Indexes: 1
- Usage
- Downloads: 33
- Abstract Views: 7
- Captures
- Readers: 12
- Mentions
- News Mentions: 1
Included in
Atmospheric Sciences Commons, Environmental Monitoring Commons, Other Computer Sciences Commons, Remote Sensing Commons
Comments
This article was originally published in IEEE Journal of Selected Topics in Applied Earth Observations and Remote Sensing, volume 17, in 2024. https://doi.org/10.1109/JSTARS.2024.3383397