Document Type
Article
Publication Date
12-21-2022
Abstract
As a crucial food crop, potatoes are highly consumed worldwide, while they are also susceptible to being infected by diverse diseases. Early detection and diagnosis can prevent the epidemic of plant diseases and raise crop yields. To this end, this study proposed a weakly-supervised learning approach for the identification of potato plant diseases. The foundation network was applied with the lightweight MobileNet V2, and to enhance the learning ability for minute lesion features, we modified the existing MobileNet-V2 architecture using the fine-tuning approach conducted by transfer learning. Then, the atrous convolution along with the SPP module was embedded into the pre-trained networks, which was followed by a hybrid attention mechanism containing channel attention and spatial attention submodules to efficiently extract high-dimensional features of plant disease images. The proposed approach outperformed other compared methods and achieved a superior performance gain. It realized an average recall rate of 91.99% for recognizing potato disease types on the publicly accessible dataset. In practical field scenarios, the proposed approach separately attained an average accuracy and specificity of 97.33% and 98.39% on the locally collected image dataset. Experimental results present a competitive performance and demonstrate the validity and feasibility of the proposed approach.
Recommended Citation
Chen, J., Deng, X., Wen, Y. et al. Weakly-supervised learning method for the recognition of potato leaf diseases. Artif Intell Rev 56, 7985–8002 (2023). https://doi.org/10.1007/s10462-022-10374-3
Copyright
Springer
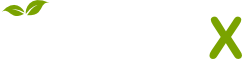
- Citations
- Citation Indexes: 27
- Usage
- Downloads: 38
- Abstract Views: 13
- Captures
- Readers: 50
- Mentions
- News Mentions: 1
Included in
Agriculture Commons, Other Electrical and Computer Engineering Commons, Other Engineering Commons, Other Food Science Commons
Comments
This is a pre-copy-editing, author-produced PDF of an article accepted for publication in Artificial Intelligence Review, volume 56, in 2023 following peer review. The final publication may differ and is available at Springer via https://doi.org/10.1007/s10462-022-10374-3.
A free-to-read copy of the final published article is available here.