Document Type
Article
Publication Date
8-17-2024
Abstract
Cycles of wildfire and rainfall produce sediment-laden floods that pose a hazard to development and may clog or overtop protective infrastructure, including debris basins and flood channels. The compound, post-fire flood hazards associated with infrastructure overtopping and clogging are challenging to estimate due to the need to account for interactions between sequences of wildfire and storm events and their impact on flood control infrastructure over time. Here we present data sources and calibration methods to estimate infrastructure clogging and channel overtopping hazards on a catchment-by-catchment basis using the Post-Fire Flood Hazard Model (PF2HazMo), a stochastic modeling approach that utilizes continuous simulation to resolve the effects of antecedent conditions and system memory. Publicly available data sources provide parameter ranges needed for stochastic modeling, and several performance measures are considered for model calibration. With application to three catchments in southern California, we show that PF2HazMo predicts the median of the simulated distribution of peak bulked flows within the 95% confidence interval of observed flows, with an order of magnitude range in bulked flow estimates depending on the performance measure used for calibration. Using infrastructure overtopping data from a post-fire wet season, we show that PF2HazMo accurately predicts the number of flood channel exceedances. Model applications to individual watersheds reveal where infrastructure is undersized to contain present-day and future overtopping hazards based on current design standards. Model limitations and sources of uncertainty are also discussed.
Recommended Citation
Jong‐Levinger, A., Houston, D., & Sanders, B. F. (2024). Estimating post‐fire flood infrastructure clogging and overtopping hazards. Water Resources Research, 60, e2023WR036522. https://doi.org/10.1029/2023WR036522
Supporting Information S1
Copyright
The authors
Creative Commons License
This work is licensed under a Creative Commons Attribution-Noncommercial-No Derivative Works 4.0 License.
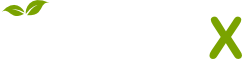
- Citations
- Citation Indexes: 1
- Usage
- Downloads: 35
- Abstract Views: 6
- Captures
- Readers: 14
- Mentions
- News Mentions: 27
Included in
Environmental Indicators and Impact Assessment Commons, Environmental Monitoring Commons
Comments
This article was originally published in Water Resources Research, volume 60, in 2024. https://doi.org/10.1029/2023WR036522