Document Type
Article
Publication Date
6-27-2019
Abstract
Despite the abundance of research on coral reef change detection, few studies have been conducted to assess the spatial generalization principles of a live coral cover classifier trained using remote sensing data from multiple locations. The aim of this study is to develop a machine learning classifier for coral dominated benthic cover-type class (CDBCTC) based on ground truth observations and Landsat images, evaluate the performance of this classifier when tested against new data, then deploy the classifier to perform CDBCTC change analysis of multiple locations. The proposed framework includes image calibration, support vector machine (SVM) training and tuning, statistical assessment of model accuracy, and temporal pixel-based image dierencing. Validation of the methodology was performed by cross-validation and train/test split using ground truth observations of benthic cover from four dierent reefs. These four locations (Palmyra Atoll, Kingman Reef, Baker Island Atoll, and Howland Island) as well as two additional locations (Kiritimati Island and Tabuaeran Island) were then evaluated for CDBCTC change detection. The in-situ training accuracy against ground truth observations for Palmyra Atoll, Kingman Reef, Baker Island Atoll, and Howland Island were 87.9%, 85.7%, 69.2%, and 82.1% respectively. The classifier attained generalized accuracy scores of 78.8%, 81.0%, 65.4%, and 67.9% for the respective locations when trained using ground truth observations from neighboring reefs and tested against the local ground truth observations of each reef. The classifier was trained using the consolidated ground truth data of all four sites and attained a cross-validated accuracy of 75.3%. The CDBCTC change detection analysis showed a decrease in CDBCTC of 32% at Palmyra Atoll, 25% at Kingman Reef, 40% at Baker Island Atoll, 25% at Howland Island, 35% at Tabuaeran Island, and 43% at Kiritimati Island. This research establishes a methodology for developing a robust classifier and the associated Controlled Parameter Cross-Validation (CPCV) process for evaluating how well the model will generalize to new data. It is an important step for improving the scientific understanding of temporal change within coral reefs around the globe.
Recommended Citation
Gapper, J.; El-Askary, H.; Linstead, E.; Piechota, T. Coral Reef Change Detection in Remote Pacific Islands Using Support Vector Machine Classifiers. Remote Sens. 2019, 11, 1525; doi:10.3390/rs11131525.
Peer Reviewed
1
Copyright
The authors
Creative Commons License
This work is licensed under a Creative Commons Attribution 4.0 License.
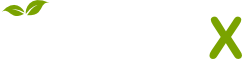
- Citations
- Citation Indexes: 45
- Usage
- Downloads: 133
- Abstract Views: 39
- Captures
- Readers: 79
- Mentions
- Blog Mentions: 1
Included in
Biology Commons, Environmental Health and Protection Commons, Environmental Indicators and Impact Assessment Commons, Environmental Monitoring Commons, Marine Biology Commons, Other Environmental Sciences Commons, Remote Sensing Commons, Terrestrial and Aquatic Ecology Commons
Comments
This article was originally published in Remote Sensing, volume 11, in 2019. DOI: 10.3390/rs11131525